Quick Summary :
From automating workflows to advanced analytics, AI and ML-driven decision-making is something every business prefers to have. Without proper Data Infrastructure Management, business dashboards would be riddled with unreliable data and hallucinated predictive modeling. In short, poor data engineering equals poor and unprofitable business decisions. On the other hand, intelligently engineered data turns chaos into clarity. Data engineering is the central force that powers scalable data architectures and helps organizations in managing complex data pipelines.
Data Engineering covers the art and science part of the process and, more importantly, lays the base right for crafting the perfect strategy for building and designing the data workflows. Data engineering consulting is the new powerhouse driving intelligent business decisions in 2025. As such, data engineers are in heavy demand to resolve chaotic situations, turn messy data formats into clean structures, and draw vital insights. Together, they help enable data flow, storage, transformation, and analysis—core pillars of modern data engineering.
So, it’s no more about moving data from point A to point B. It’s about elevating it, making it faster, smarter, and clearer for top decision-makers in a business.
That’s why today’s systems are more focused on managing structured, semi-structured, and unstructured data at petabyte scales, aligning closely with Big Data demands.
With Data Engineering Tools like Apache Hadoop, Apache Spark, Kafka, and Apache Airflow, businesses have realized the difference between “nice to have” options and essentials.
Here’s a complete guide on data engineering in 2025 that will help take your business to new heights. Keep reading!
Fundamentals of Data Engineering: What Every Business Must Know
Data engineering creates a solid foundation for analytics, automation, and AI initiatives. None of these will work properly if there is no precision data engineering. Modern data engineering isn’t just about tools or coding; it is the development of a system that ensures all data is clean and accessible, and data is stored safely and governed using ethical means. Compliance is a key part of a perfect data engineering strategy.
Without the right data engineering models or SOPs, even the smartest AI models or most expensive BI tools will crumble under poor data quality. In fact, global businesses lose $15 million per organization per annum just because of poor data quality. In short, data engineering is the base that helps businesses build pipelines that support structured, semi-structured, and unstructured data. All analytics and operational automation are built with data engineering.
Latency Control: Ensure both batch and real-time processing capabilities to meet different use cases. | Security First: Bake in encryption, access control, and audit trails from day one. |
Data Pipeline Design: Focus on modular and reusable data flows that are easy to debug and scale. | Schema Management: Define, validate, and version schemas to prevent breaking changes. |
Why Data Engineering Matters in 2025?
Global data volume is expected to grow by unimaginable numbers. According to industry reports, worldwide data creation volume is anticipated to surpass 394 zettabytes by 2028—driven heavily by the explosive growth of Data Science and Data Integration. The global data engineering as a service (DaaS) market is surging at a whopping CAGR of 22.8%.
Every app notification, social media scroll, IoT sensor clip, and online transaction contributes to this data deluge. As a result, businesses that aren’t ready to invest in robust data engineering consulting will find themselves falling behind fast.
Despite the rapid evolution of AI, data engineering is still largely dependent on clean, structured, accessible data supported by strong Data Governance frameworks. To make things happen, you need skilled data engineers who can build and automate reliable data pipelines, which are one of the top data engineering best practices today.
For companies overwhelmed by chaotic data, partnering with expert data engineering consulting firms ensures turbocharged pipelines and scalable Data Infrastructure aligned to the fundamentals of data engineering.
This directly translates into:
- Faster and accurate decision-making
- Smooth customer engagement and personalization
- Real-time optimization for logistics and supply chains
- Data-driven product development based on actual user behavior
Market studies show over 90% of data teams operating at or beyond capacity. That means, there’s no better time than now to modernize Enterprise Data Architecture to prevent breakdowns and data chaos.
How Leading Industries Are Betting Big on Data Engineering?

Data engineering is no longer confined to just tech companies. Every industry that touches people, machines, or money is now in the thick of Big Data Engineering.
1. Finance
Banks, fintechs, stock exchanges, and insurance companies are equally obsessed with Data Pipeline Automation Trends. With millions of transactions every second, sophisticated fraud detection and data warehousing are no longer optional luxuries. Advanced data modeling combined with resilient infrastructures powers these real-time systems. No wonder businesses are fast embracing sophisticated fraud detection systems to flag suspicious activities midway.
But the bigger picture goes well beyond fraud. It’s got to do with real-time risk analysis, regulatory reporting, and personalized financial services. These things are only made possible by advanced and resilient data architectures. This is why top investment banks are now processing petabytes of financial market data daily, powering trading algorithms. Imagine a 30-second delay, which could translate to millions lost.
2. Retail
Gut-based marketing has no space in 2025. As a retailer, if you aren’t paying attention to your customer data, then what are you even doing? With real-time data streams, you have the power to track everything from warehouse stocks to customer clicks. Thus, the need of the hour is dynamic adjustments for:
- Product placements
- Marketing offers
- Pricing models
Modern-day retailers are bent on harnessing the power of hyper-personalized marketing, relying on micro-behavior for what your customers glanced at versus what they actually bought. The approach has taken “guesswork” out of the equation and has laser-targeted ad campaigns spearheaded by predictive modeling with clean and real-time data. For instance, some brands throw flash discounts when the customer stands in person in-store. That’s IoT sensors and mobile app trackers doing their magic.
Unify your sources with intelligent pipelines and enterprise-grade data infrastructure.
3. Healthcare
Healthcare is one such domain where data engineering decides the fate of life. Whether it’s integrating patient records, tracking genomic data, or from diagnostic imaging to fitness tracker stats, every bit contributes towards a privacy complaint and a unified data ecosystem. With stringent regulations like HIPAA focused on patient privacy, data governance is no longer an option.
One of the best instances in this case is precision medicine, which allows tailored treatment delivery for each patient. Thanks to seamless integration and transformation of massive datasets, allowing robust pipelines to:
- Help doctors receive lab results faster
- Diagnose early symptoms and detect medical conditions from imaging data
- Using predictive analysis to optimize staff allocation, especially during emergencies
4. Manufacturing
Modern-day factories are powered by big data engineering and are all about speed, smartness, and automation. And thanks to big data pipelines and IoT, things are happening. Machine sensors offer access to a truckload of data like pressure, temperature, vibrations, and more. Real-time analysis of such data helps predict equipment failures even before they happen, also known as predictive maintenance. Thus, data engineering in manufacturing helps with :
- Reduced downtime
- Extension of machinery lifespan
- Slashed operational costs
5. Transportation and Logistics
Fleet management today isn’t just about GPS coordinates. It’s about full-scale data orchestration that factors in traffic conditions, fuel consumption, delivery delays, driver performance, and more, often in real time. Data engineering plays a pivotal role in optimizing supply chains and transportation networks. The rise of autonomous vehicles and smart routing algorithms has made scalable, cloud-powered data pipelines a necessity. Logistics giants deploy predictive analytics and data integration for route planning and live tracking.
Here’s where data engineering makes an impact:
- Real-time vehicle tracking and fuel efficiency analysis
- Predictive route optimization based on traffic and weather
- Sensor data integration for predictive maintenance
- Dynamic ETA calculation and delivery automation
6. Media and Entertainment
From Netflix to Spotify, who doesn’t like “Recommended for You” lists? Besides entertainment, they help companies analyze social media trends, viewer behavior, and content consumption patterns. The magic workers behind it are data science processing and data lake management. The results help companies dynamically adjust:
- When the recommendations are best shown
- What kind of recommendations need to be shown
- How the recommendations are to be marketed best
7. Energy
The world has gradually embraced the use of smart energy grids and renewables. Data engineering is the ultimate backbone for devices like smart meters, solar panels, electric vehicles, and grid sensors. Together, they are trusted sources for continuous telemetry data streams using cloud data engineering. This, in turn, helps companies:
- Seamlessly predict energy demand spikes
- Prevent unwanted blackouts
- Optimize grid loads
- Trade surplus energy across open markets
8. Education and EdTech
From LMS platforms to virtual classrooms, every click, quiz score, or study session is a data point waiting to be transformed into insights. Data engineering is now the backbone of modern education platforms that scale to millions of students globally. Institutions and EdTech companies are relying on real-time analytics and structured feedback systems.
Where data engineering is changing the education game:
- Real-time performance analytics for adaptive learning
- Personalized content recommendations using student behavior data
- Integration of multiple systems (LMS, CMS, ERP) for unified reporting Predictive modeling to detect at-risk students and improve retention
Top Data Engineering Tools Making Waves in 2025
Gone are the days when a handful of tools were enough. In 2025, the data engineering toolbox is diverse, AI-integrated, and cloud-optimized. Picking the right tools can mean the difference between a high-performing pipeline and a bottlenecked nightmare. Each stage of the pipeline—from ingestion to orchestration—has tools specifically built for speed, flexibility, and collaboration.
With data teams stretched to their limits, automation and intelligent tools are helping scale operations efficiently. Many of these tools are also enabling non-engineers to participate in data workflows through intuitive UIs and low-code setups.
Must-Have Tools in Your Stack:
Ingestion | T-ingestor, Apache Kafka, Fivetran, Airbyte for streaming and third-party data integration. |
Transformation | dbt for modular SQL transformations and lineage tracking. |
Orchestration | Apache Airflow, Prefect, and Dagster to manage dependencies and schedules. |
Storage | Databricks Lakehouse, Sancus, BigQuery, Snowflake for modern hybrid warehousing. |
Visualization | Power BI, Tableau, T-Voyager |
How To Build a Rock-Solid Infrastructure in 2025 With Data Engineering?
No matter how flashy your AI models look, data engineering sets the foundation for saving money and getting the job done right. But in 2025, the fundamentals of data engineering have transformed into something bigger and more powerful. Today, it’s not just about shifting from Point A to B, but it’s about moving at scale, in real time, and still keeping it compliant and competitive.
So, what makes a successful data engineering process? The answer is a structured and flexible lifecycle. You see, it’s never a one-size-fits-all thong. The details are bound to vary depending on data sources, industry, compliance requirements, and future organizations’ goals. But the core pillars will always remain the same. You need usable, actionable data to master every stage.
Here’s an exclusive blueprint to help you get started.
- Data Ingestion is where you capture data from different sources like databases, IoT devices, and external third-party data feeds.
- Data integration and transformation involves cleaning, normalizing, reshaping, and deduplication. The gold standard in this case was ETL (Extract, Transform, Load), but with cloud scaling, ELT (Extract, Load, Transform) has now taken the driver’s seat.
- Data Storage, especially for Structured data, uses warehouses like Snowflake, Redshift, and BigQuery. For semi-structured and unstructured data, work with data lakes like Azure Data Lake, AWS S3, or a hybrid option like Databricks Lakehouse. Remember, storage doesn’t mean dumping.
- Data Governance is a must-have feature in 2025 to facilitate lineage tracking, metadata management, and RBAC.
- (Role-based access control), and keep it compliant with leading regulations like CCPA, GDPR, HIPAA, and AI ethics policies.
Following the above-mentioned steps to build data infrastructure discussed will help you steer clear of mishandling data. After all, when a company is accused of data mishandling, it’s a costly mistake. You don’t just end up paying fines, but in the process, you lose customer trust, brand equity, and company talent.
To make life easy, we’ve further got you covered with some Data Engineering Best Practices to build a rock-solid data infrastructure:
- Start by defining your key business goals, KPIs, and other needs upfront. Ditch the “collect everything and sort later” stage to keep things functional.
- Always make room to architect scalable platforms. This is usually done by combining lakehouses and warehouses.
- Next, set up robust ingestion pipelines capable of handling both historical and real-time data.
- Invest time and resources to build flexible, modular transformation layers. (Pro Tip: Frameworks like dbt and orchestration tools like Airflow make great options)
- Pick efficient storage formats like ORC, Parquet, or Avro to maximize performance.
- Ensure strong governance comprising encryption, audit trails, access policies, and masking.
- Monitor, alert, and automate pipeline health. Some of the best observability tools include Monte Carlo, Databand, and Great Expectations.
Switch to real-time analytics and cloud-native data architectures tailored for your enterprise.
Data Engineering Best Practices in 2025
As data grows more complex, following best practices is no longer optional—it’s critical to survival. Today’s engineering teams must build infrastructure that’s scalable, observable, and governed from end to end. Whether it’s working with terabytes or petabytes, the goal remains the same: enable fast, accurate, and trustworthy insights.
These best practices are universal guardrails that help avoid data silos, prevent operational headaches, and promote organizational data literacy. Think of them as your secret sauce to scale without breaking down.
• Modular Architecturel: Design pipelines in components that can be independently developed and tested. | • Version Control & CI/CD: Treat data workflows like software code using Git-based deployments. |
• Data Observability: FocusContinuously monitor data quality and pipeline health using tools like Monte Carlo or Great Expectations. | •Cost Optimization:Choose storage and compute wisely (columnar formats, query pruning, cloud-tiered storage). |
From development and deployment, data engineering best practices help create the right systems. As per the Modern Data Engineering Playbook by Thoughtworks, these practices include:
- Test-driven development
- Pair programming
- Trunk-based development
- Build security in
- Automated deployment pipeline
- Fast automated build
- Build for production
Future Trends In Data Engineering For 2025 And Beyond
2025 could be the renaissance for modern data engineering, considering the faster, smarter, and self-healing data pipelines. They are integrated deeply into almost every other business today. And that’s why it makes it even more challenging to accelerate innovation. The big data engineering services market size is projected to reach US dollars 187.19 billion by 2030. It is currently growing at a CAGR of 15.38%.
Whether you’re an established business or a startup, you will need to embrace cloud-native architectures, real-time streaming, AI-assisted development, and decentralized data ownership models. Here’s a closer look at some of the best modern-day data engineering trends for 2025 and beyond.
- Making everything cloud-native: Forget lift-and-shift old systems and start rebuilding natively with services like AWS Glue, BigQuery, and Azure Synapse.
- Working with real-time data pipelines: Kinesis, Kafka, and Flink have become standard issues across data pipelines. And while batch processing still exists, it isn’t much help in today’s scenario, where you need to solve issues before they get escalated.
- DataOps and CI/CD Pipelines: This is like DevOps but strictly for pipelines consisting of monitoring, automated testing, and subsequent deployment of data workflows, minus downtime.
- AI-Augmented ETL: The best option when you’re looking for auto-schema suggestions, self-healing pipelines, and intelligent anomaly detection. Think of AI as your junior data engineer helping you accelerate things.
- Data Mesh & Data Fabric: With each business wanting to own its product, centralized lakes are becoming obsolete. That’s where data mesh and fabric come in with a decentralized approach, making things faster and a lot less bureaucratic.
- Low-Code/No-Code Data Tools: With the rise in Citizen data engineer roles, businesses are bent on building basic pipelines with nothing but drag-and-drop features. Advanced tools like Airbyte, Talend, Fivetran, and Matillion have already shown the way.
Get Ready To Embrace A Future That Belongs to the Data-Ready
So, you see how data engineering is changing the game forever. And by the end of 2025, businesses will not only be winning the show with data but will practically live and breathe data. So, there’s no better time than now to partner with a reliable data analytics company that can help you accelerate innovation. From building profitable strategies to elevating customer relationships, it all boils down to having cleaner, faster, and smarter data pipelines.
This is where you need a data engineering consulting firm, not only to act as a service provider but as a strategic partner, reshaping the digital backbone of your business.
At X-Byte, we’ve come a long way, helping forward-looking businesses bend the curve with our full-scale data engineering solutions. From data analytics consulting to data infrastructure management, we are right at the center of building a futuristic Enterprise Data Architecture.
Are you a new or existing business looking to scale with data engineering and make an impact? We would be happy to discuss the scope of work and help you chalk out the best solution
Best Practices To Effectively Implement Supply Chain Predictive Analytics
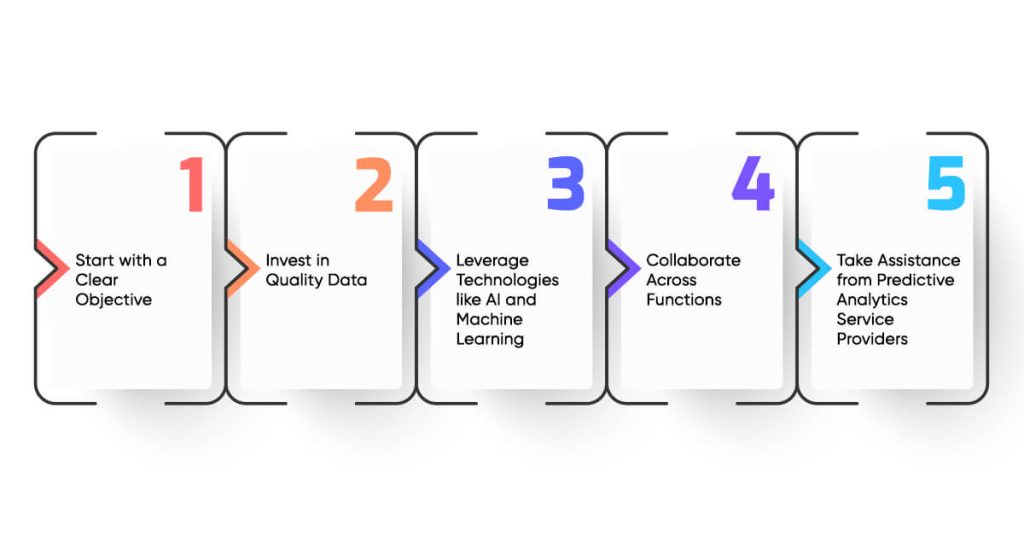
A strategic and scalable approach is necessary for implementing supply chain analytics. The following best practices ensure successful deployment of data analytics in supply chain. Let’s have a look.
1. Start with a Clear Objective
Organizations need to establish particular measurable goals that identify supply chain problems before beginning predictive analytics work. Organizations benefit from focused goals as they establish clear directions that enable them to evaluate performance, reduce costs, and make better demand predictions. The establishment of clear goals regulates the entire data collection and model development procedure to achieve alignment between analytic initiatives and general business targets.
2. Invest in Quality Data
For successful predictive analytics to occur, one needs high-quality data since it serves as the foundation. Businesses must focus on obtaining precise and uniform data from the entire supply chain interaction points. The analytics depend on ERP and CRM systems within the organization, along with external sources that include supplier and customer data. The analysis becomes actionable and completely synergized with actual conditions when organizations integrate their complete information sources.
3. Leverage Technologies like AI and Machine Learning
AI systems and machine learning models generate automated complex data analysis that enables them to discover scalable trends, relationships, and spot anomalous patterns. The systems learn progressively from fresh data, which enhances their ability to forecast and make decisions. Business organizations employing AI models in their demand planning processes, inventory management, and risk detection frameworks achieve greater adaptability for dealing with supply chain challenges.
4. Collaborate Across Functions
Supply chain predictive analytics is separate from being an IT initiative, as it needs departmental collaboration across operations. Operational groups with logistics personnel and procurement staff, along with IT teams, should define necessary data requirements while interpreting results and implementing findings. Organizations determine better practical outcomes when they integrate predictive analytics across different functional teams because this integration leads to embedded analytical workflows that improve effectiveness as well as maintenance sustainability.
5. Take Assistance from Predictive Analytics Service Providers
The adoption of predictive analytics within organizations becomes faster when top-quality predictive analytics consulting firms are partners. Consultants with deep industry expertise, proven methodologies, and technical expertise aid organizations as they navigate typical operational challenges. The integration of consultants helps organizations conduct change management while building system connections and delivering training processes to create a seamless transition to data-based supply chain operations that provide concrete performance outcomes.
Lacking the specialized expertise needed to implement advanced predictive models? Leverage X-Byte's team of supply chain analytics experts to deploy custom solutions!
Use Cases of Supply Chain Predictive Analytics
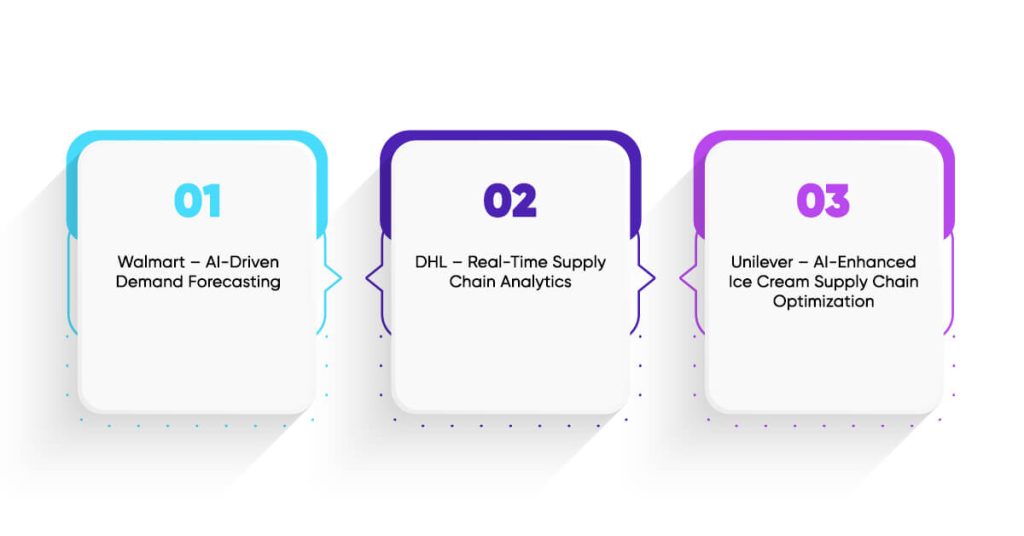
1. Walmart – AI-Driven Demand Forecasting
AI and machine learning help Walmart handle its inventory management requirements more effectively during busy periods for shopping. Walmart uses an AI-based software to examine historical sales records and search trends, together with weather reports and market statistics from nearby locations, as it positions products in both its distribution facilities and retail locations. The method allows people to locate their required products at the exact time they seek them, contributing to better shopping conditions and operational performance.
2. DHL – Real-Time Supply Chain Analytics
The Resilience360 platform of DHL uses weather tracking technology with predictive analytics to reduce the impact on supply chain operations due to severe weather occurrences. The system evaluates upcoming hurricane and cyclone movement paths to give alerts on the effects of these storms on supply chain networks. The system enables businesses to send shipments on different routes and adapt their logistics activities before severe weather impacts service delivery
3. Unilever – AI-Enhanced Ice Cream Supply Chain Optimization
The ice cream supply chain of Unilever consists of 60 countries and 35 factories alongside approximately 3 million freezer cabinets. This supply chain has become seamless because of the use of artificial intelligence (AI) and digital tools.
Unilever uses AI systems that evaluate weather patterns, consumer trends, and sales history to calculate precise demand projections. When implemented in Sweden, the prediction accuracy increased by 10%, thus enabling better manufacturing schedules and, at the same time, diminishing waste occurrences. Retail orders, along with sales, have experienced a 30% boost because of data collected from 100,000 AI-powered freezers, thus proving that AI bolsters supply chain speed and operational competencies.
Conclusion
Predictive analytics in supply chain management emerges as an essential competitive factor because supply chains have become more complex, while customer demands are also constantly increasing. The combination of supply chain predictive analytics best practices and the resolution of main challenges generates a substantial business value and operational resiliency for the future.
Our Supply Chain Predictive Analytics Consulting can help you modernize your supply chain systems with advanced data analytics solutions. Our team of expert professionals assists businesses in optimizing their data through predictive analytics solutions for developing a smarter and resilient supply chain.
Make Your Supply Chain Smarter and Advanced! Explore Our Predictive Analytics Solutions!
Frequently Asked Questions
What exactly is data engineering, and how is it different from data science?
Why has data engineering become so critical in 2025?
Extensive data volumes in 2025 and the usage of data for decision-making make data engineering a proven solution for dealing with complex data sources and building reliable data pipelines. Without data engineering, businesses will have to deal with unrealistic analytics.
What are the core components of modern data engineering?
Modern data engineering includes data ingestion, transformation, storage, governance, and orchestration. These approaches are based on real-time data processing and self-healing systems.
What's the ROI timeline for investing in data engineering?
The returns for data engineering investments are visible right from the first six months. However, you get the real benefits from the next year onwards. Companies see faster analytics, lower cloud costs, improved data pipelines, and many more tangible and intangible ROIs from the investment in data engineering.
How do small and medium businesses approach data engineering without massive budgets?
SMBs can adopt modular, cloud-native solutions that scale with needs. Data engineering tools and managed services provide data engineering capabilities affordably.
Which data storage solution is best: data warehouse, data lake, or lakehouse?
Data warehouses excel at structured analytics, data lakes offer raw data flexibility, and lakehouses blend both approaches. The choice depends on your business needs. For more details, check our comparison guide here
What are the biggest challenges facing data engineering teams in 2025?
Key challenges include managing vast data volumes (peta bytes and tera bytes), growth, implementing the right governance models, breaking down silos, and finding data engineering consulting partners to build future-proof systems.
How is edge computing changing traditional data engineering paradigms?
Edge computing processes data near its source, enabling IoT applications while reducing latency. Industries like manufacturing and autonomous vehicles lead this transformation.